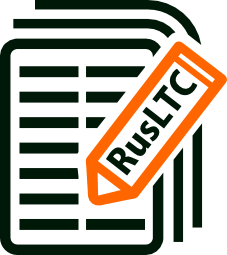
Russian Learner Translator Corpus (RusLTC) is a bi-directional multiple corpus, which stores English-Russian translations done by university translation students.
The project was initiated in 2011 by enthusiasts from the Translation Studies Department, University of Tyumen (Russia) and enjoyed support from computational linguists with the National Research University Higher School of Economics (Moscow, Russia). It has been unveiled as a fully functional online resource in 2014 at Text. Speech. Dialogue conference in Brno. In 2017 a research proposal partially based on this corpus data received support from the Russian Foundation for Basic Research (RFBR), which gave the project a boost and helped to continue making it publicly available.
Russian
RusLTC includes translations collected from 14 Russian universities, which offer specialist, BA and MA translation programmes. Most translations are produced as part of their routine and exam assignments by (often senior) students in these programmes. There are, however, translations that were submitted to translation contests held by the partner universities, translations from trainees who stu
dy translation as a supplementary professional course or have chosen translation as their second higher education (part-time and continuous learning programmes). These student populations and levels of study are reflected in the searchable corpus metadata. Translations and metadata are anonymised.
As of October 16, 2018 RusLTC counts more than 2.3 mln word tokens in total. The English-Russian subcorpus has multiple translations to 402 sources, while Russian-English part includes 125 source-targets sets. The number of translations to a single source averages at 8 (min=1, max=67). Many texts are translations of excerpts from larger sources; the median lengths of translations in the English-to-Russian subcorpus is 380 words. Detailed and automatically updated statistics.
RusLTC is aligned at sentence-level with LF Aligner; the errors in its output are corrected manually. Since September 2018 the query interface is based on a customized version of Arkhangelskiy's Tsakorpus. It supports lexical and PoS search for both sources and targets and returns sentences with the query item along with their targets/sources. The query results can be filtered by 10 metadata fields, including the translator’s gender and affiliation, education type and level, grade for the translation, year and conditions of translation (routine/exam; home/classroom) and source text genre. RusLTC includes texts in 10 genres: academic, informational, educational, essay, technical, fiction, educational, speech, letters, advertisement – see the figure on the right for their distribution). Give it a try! Explore renditions of the challenging lemmas lacking immediate counterparts such as overqualified
or lock-in
. See how weird analytical passives can get in translated Russian.
RusLTC has an associated translation error annotation project, which uses brat text annotation framework (Stenetorp et al 2012). The annotator follows a pre-defined error typology of 30 hierarchical tags, including good_solution
and note
(see the typology description and annotation guidelines (in Russian)). As of October 2018 we have annotated 765 translations, including 599 translations (230 K tokens) to 72 sources in English-to-Russian direction. The annotation environment is in everyday classroom use, and this data is growing each year.
RusLTC is downloadable as a customized TMX-file and a plaintext archive.
The corpus structure is based on the following filename conventions. Each text in the corpus receives a unique name starting with the source language code (EN or RU), the number indicating the contributor, the unique number of the source and (in case of translations) the number of an individual translation for the same source. For example, RU_1_35.txt and EN_1_35_3.txt are a Russian source and its third English translation respectively. If a source has only one translation, it still receives number 1 after the third underscore in the filename to identify that it is a translation.
We use separate header and annotation files to store metadata and error annotations. For example, RU_1_35.head.txt is a file containing metadata for the respective text; EN_1_35_3.ann is a file containing the error annotation. The current error statistics is shown in the figure to the left.
We stick to open knowledge philosophy in choosing corpus-building technology, and are happy to make the Corpus available online. To the best of our knowledge RusLTC is the third multiple learner translator corpus available online after MeLLANGE LTC (see Table 1 which outlines LTC-related research as of March 2014).
Except for its direct classroom applications, RusLTC is used as a source of data for research in Translation Studies, primarily dealing with linguistic properties of translations, translation quality assessment, variation in translation and translational competence.
Feel free to contact us at mkunilovskaya@gmail.com.
The corpus content is available under the Creative Commons Attribution-ShareAlike license. You can use it freely as long as you make the reference to the authors (Kunilovskaya, Kutuzov 2014 in the References below), and if you remix, transform, or build upon the material, you must distribute your contributions under the same license as the original.
If you are the author of any translations included in RusLTC and don’t want them to be freely available for research purposes anymore, contact the RusLTC team, and we will remove them from the corpus.
Download RusLTC texts as a plain text archive (including metadata)
Download RusLTC as an aligned TMX file
Download RusLTC description (source text title, genre, size and number of translations available, tsv)
Datasets with quality labels and scores:
Parallel documents with binary quality and professionalism labels, and comparable non-translations (docs-labels-ref-feats.tsv.gz)
Parallel documents with quality scores from error annotation (unweighted) and from DA (docs-scores_err553-unweighted_da140-2raters.tsv.gz)
Parallel sentences with quality scores from error annotation (unweighted) and from DA (sent-scores_err12369-unweighted_da3224-2raters.tsv.gz)
Back to Query interface
RusLTC data was used in a number of research projects, including corpus-based undergraduate research and much is planned for the future.
Some of the publications based on RusLTC can be found below:
- Kunilovskaya, M., Ilyushchenya, T., Morgoun, N., & Mitkov, R. (June, 2022). Source language difficulties in learner translation: Evidence from an error-annotated corpus. Target. https://doi.org/10.1075/target.20189.kun
- Kunilovskaya, M., Lapshinova-Koltunski, E., & Mitkov, R. (2021). Translationese in Russian Literary Texts. Proceedings of the 5th Joint SIGHUM Workshop on Computational Linguistics for Cultural Heritage, Social Sciences, Humanities and Literature. EMNLP, pp. 101–112.
- Kunilovskaya, M. & G. Corpas Pastor (2021). Translationese and register variation in English-to-Russian professional translation. In L. Lim, D. Li, and V. Wang (Eds.), New Perspectives on Corpus Translation Studies. Springer.
- Kunilovskaya, M., & Lapshinova-Koltunski, E. (2020). Lexicogrammatic translationese across two targets and competence levels. In N. Calzolari, F. Bechet, P. Blache, K. Choukri, C. Cieri, T. Declerck, S. Goggi, H. Isahara, B. Maegaard, J. Mariani, & A. Others (Eds.), Proceedings of the 12th Conference on Language Resources and Evaluation (LREC 2020) (pp. 4102–4112). The European Language Resources Association (ELRA).
- Kunilovskaya, M., & Lapshinova-Koltunski, E. (2019). Translationese features as indicators of quality in English-Russian human translation. In I. Temnikova, C. Orasan, G. Corpas Pastor & R. Mitkov (Eds.), Proceedings of the 2nd Workshop on Human-Informed Translation and Interpreting Technology (HiT-IT 2019) (pp. 47–56). INCOMA Ltd. http://dx.doi.org/10.26615/issn.2683-0078.2019_006
- A poster presenting RusLTC 2.0 at Artificial Intelligence and Natural Language Conference (AINL 2018)
- Kunilovskaya, M., Morgoun N. & Pariy A. (2018). Learner vs. professional translations into Russian: Lexical profiles. Translation and Interpreting (ISSN 1836-9324), 10(1), 33-52. https://doi.org/10.12807/ti.110201.2018.a03
- Kunilovskaya, M. & Morgoun N. (2018). Do translation textbooks address real-life translation problems: evidence from corpus-based error analysis. In Book of abstracts 13th Teaching and Language Corpora Conference, (TALC 2018). Faculty of Education, University of Cambridge, 102-103 See slides
- Kunilovskaya M. & Kutuzov A. (2018) Universal Dependencies-based syntactic features in detecting human translation varieties Proceedings of the 16th International Workshop on Treebanks and Linguistic Theories (pp. 27-35). Association for Computational Linguistics.
- Kunilovskaya, M. A., Ilyushchenya, T. A., & Kovyazina, M. A. (2017). Corpus-based quality assessment of error-tagged learner translations. Tyumen State University Herald. Humanities Research. Humanitates, 3(3), 94–112. https://doi.org/10.21684/2411-197X-2017-3-3-94-112 (in Russian)
- Kunilovskaya M. & Kutuzov A. (2017) Testing target text cohesion: machine learning approach to modeling sentence boundaries in English-Russian translationNew perspectives on cohesion and coherence: implications for translation. Edited by: Kerstin Kunz, Ekaterina Lapshinova-Koltunski & Katrin Menzel. Language Science Press, 2017 (pp. 75−103).
- Kunilovskaya M. (2017) Linguistic tendencies in English to Russian translation: the case of connectives. In Computational Linguistics and Intellectual Technologies: Proceedings of the International Conference
Dialogue 2017
(pp. 221-233) Appendix 1. Semantic taxonomy of connectives
- Kunilovskaya M. & Kutuzov A. (2015) A quantitative study of translational Russian (based on а translational learner corpus)// Труды международной конференции
Корпусная лингвистика-2015
. – Спб.: С.-Петербургский гос. университет, Филологический факультет, 2015. – С. 33–40.
- Kunilovskaya M.(2015) How Far do we Agree on the Quality of Translation?In English Studies at NBU, 2015, Vol. 1, Issue 1, 18–31.
- Kunilovskaya, M., Kutuzov A. (2014) Russian Learner Translator Corpus: Design, research potential and applications In Text, Speech and Dialogue (pp. 315-323). Springer International Publishing.
- Kunilovskaya, M.A. (2013) Error-based TQA and Error Mark-up in BRAT. In Proceedings of International Conference on Translatology, Problems of Translation and Methods of Teaching Translation. Nizhny Novgorod, Russia. Issue 16. Vol 1, 59–71 (in Russian).
[Куниловская, М.А. Классификация переводческих ошибок и их электронная разметка в brat //Проблемы теории, практики и дидактики перевода: Сборник научных трудов. Серия "Язык. Культура. Коммуникация". Выпуск 16. Том 1. - Нижний Новгород: Нижегородский государственный лингвистический университет им. Н.А. Добролюбова, 2013. - С. 59–71].
- Kunilovskaya, M.A. and Morgoun, N.L. (2013) Gains And Pitfalls Of Sentence-Splitting.In Translation In Perm National Research Polytechnic University Herald / Linguistic and Pedagogy. 8 (50), 152–166.
- Ilyushchenya, T.A. and Kunilovskaya, M.A. (2013) Inter-rater Reliability in Student Translation Evaluation. In Proceedings of International Conference on Translation Studies Ecology of Translation: Interdisciplinary Research and Perspectives. OCTOBER 4-5, Tyumen, Russia, 105–115 (in Russian).
[Ильющеня, Т.А, Куниловская, М.А. (2013). Надежность результатов описания и оценки качества учебного письменного перевода // Экология перевода: перспективы междисциплинарных исследований: материалы I Международной научно-практической конференции (г. Тюмень, 4-5 октября 2013 г.)". – Тюмень: Издательство «ШУКЛИН & АЛЕКСАНДРОВ» 2013. – С. 105–115].
- Kunilovskaya, M.A. (2012) Towards Translation Error Mark-up in Russian Learner Translator Corpus.Paper presented at Translation Forum Russia, September 30. Kazan, Russia [Куниловская, М.А. Классификация переводческих ошибок для создания разметки в учебном параллельном корпусе Russian Learner Translator Corpus, in Lingua mobilis: Научный журнал, № 40. – ЧелГУ: Лаборатория межкультурных коммуникаций, 2013. – С. 141–159].
- Kutuzov, A.B., Kunilovskaya M.A., Oschepkov A.Yu. and Chepurkova A., Yu. (2012) Russian Learner Parallel Corpus as a Tool for Translation Studies. In Computational Linguistics and Intellectual Technologies. Papers from the Annual International Conference
Dialogue
, Issue 11. Vol 1 of 2, 362–369.
- Kutuzov, A.B. (2012) Is there a difference between male and female translations (based on the RusLTC data). In Proceedings of International Conference on Translatology, Problems of Translation and Methods of Teaching Translation. Nizhny Novgorod, Russia. Issue 15. Vol 1, 97–104 (in Russian).
[Кутузов, А.Б. Переводы мужские и женские: есть ли разница (на материале Корпуса несовершенных переводов)? // Проблемы перевода, лингвистики и литературы: сборник научных трудов. Серия "Язык. Культура. Коммуникация". Выпуск 15. Том 1. - Нижний Новгород: НГЛУ, 2012. – C. 97–104].
- Kutuzov, A.B. (2011) Russian Learner Translator Corpus: Importance of the Project. In Proceedings of International Conference on Translatology, Problems of Translation and Methods of Teaching Translation. Nizhny Novgorod, Russia. Issue 14. Vol 1 (in Russian).
[Кутузов, А.Б. Корпус несовершенных переводов: необходимость проекта // Сборник научных трудов "Проблемы теории, практики и дидактики перевода", вып.14, т.1. - Нижний Новгород, 2011].

Russian Learner Translator Corpus by RusLTC Team is licensed under a Creative Commons Attribution-ShareAlike 4.0 International License.
Permissions beyond the scope of this license may be available at rlpcorpus@gmail.com.